With the imminent demise of Google Analytics Universal in July 2023 I’ve been searching for an alternative and found an Open Source project from Plausible.io. Slight warning, this post is largely for myself to remember what I did to get this going so YMMV.
I host a few sites on Amazon Lightsail and while I found a few great posts discussing self-hosting of Plausible I found these setups perhaps a bit more complicated that I think it needed to be. For example, Lightsail has instances that include both a web server and Bitnami’s Let’s Encrypt SSL tool already installed so starting from an OS-only instance type requires some unnecessary configuration.
To get started quickly browse to the Amazon Lightsail console and under Apps + OS select the NodeJS instance using Debian (as of this writing Feb 2023). You could use and “OS Only” option but then you’d be left installing a number of packages vs. using this pre-built instance.
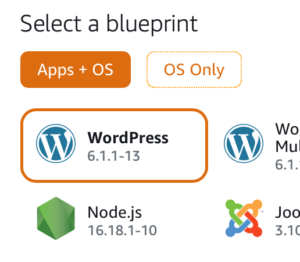
You’ll want to setup a static IP address for this instance for your DNS entry (see below). Next, SSH into your instance and create an “apps” folder under /opt/bitnami:
$ cd /opt/bitnami
$ sudo mkdir apps
$ cd apps
Clone the Plausible repo and setup the configuration as per the great Plausible self-hosting guide:
$ sudo git clone https://github.com/plausible/hosting plausible
$ cd plausible
# Generate SECRET_KEY_BASE value using openssl
$ openssl rand 64 | base64 -w 0 ; echo
$ sudo vi plausible-conf.env
# Edit the plausible config file and add config values
Install a missing gpg package:
$ sudo apt update -y
$ sudo apt-get install gpg
$ sudo curl -fsSL https://download.docker.com/linux/ubuntu/gpg | sudo gpg --dearmor -o /usr/share/keyrings/docker-archive-keyring.gpg
$ echo "deb [arch=amd64 signed-by=/usr/share/keyrings/docker-archive-keyring.gpg] https://download.docker.com/linux/ubuntu focal stable" | sudo tee /etc/apt/sources.list.d/docker.list > /dev/null
$ sudo apt-get update
$ sudo apt-get install docker-ce docker-ce-cli docker-compose containerd.io -y
There are only a few config values you need to launch your instance and start tracking your site’s analytics:
ADMIN_USER_EMAIL=
ADMIN_USER_NAME=
ADMIN_USER_PWD=
BASE_URL=
SECRET_KEY_BASE=
NOTE: I added DISABLE_REGISTRATION
as I’m the only user who needs access.
I use Cloudflare for my CDN/DNS and I created an A NAME DNS-only entry pointing to analytics.<domain>.com which I use for Plausible.
Next, again following the self-hosting guide I started up the server using docker-compose:
$ sudo docker-compose up -d
The docs weren’t super clear about getting the first user created but browse to your URL and register the first user directly on the site. Following that I would recommend disabling registrations in the plausible-conf.env
using:
DISABLE_REGISTRATION=true
Next, run Bitnami’s bncert-tool and setup a Let’s Encrypt SSL cert for your server once you’ve made a donation.
Finally, setup your Apache virtual host config by copying the existing sample HTTPS configuration:
$ cd /opt/bitnami/apache/conf/vhosts
$ sudo cp sample-https-vhost.conf.disabled plausible-https-vhost.conf
Here’s my Apache vhost file for Plausible (using X-Robots-Tag to prevent crawling):
<VirtualHost 127.0.0.1:443 _default_:443>
ServerName analytics.<domain>.com
ServerAlias *
SSLEngine on
Header Set X-Robots-Tag "noindex, noarchive, nosnippet"
SSLCertificateFile "/opt/bitnami/apache/conf/analytics.<domain>.com.crt"
SSLCertificateKeyFile "/opt/bitnami/apache/conf/analytics.<domain>.com.key"
DocumentRoot /opt/bitnami/apps/plausible
# BEGIN: Configuration for letsencrypt
Include "/opt/bitnami/apps/letsencrypt/conf/httpd-prefix.conf"
# END: Configuration for letsencrypt
# BEGIN: Support domain renewal when using mod_proxy without Location
<IfModule mod_proxy.c>
ProxyPass /.well-known !
</IfModule>
# END: Support domain renewal when using mod_proxy without Location
<Directory "/opt/bitnami/apps/plausible">
Options -Indexes +FollowSymLinks -MultiViews
AllowOverride All
Require all granted
</Directory>
ProxyPass / http://localhost:8000/
ProxyPassReverse / http://localhost:8000/
# BEGIN: Support domain renewal when using mod_proxy within Location
<Location /.well-known>
<IfModule mod_proxy.c>
ProxyPass !
</IfModule>
</Location>
# END: Support domain renewal when using mod_proxy within Location
</VirtualHost>
Following this suggestion, in /opt/bitnami/apps/plausible
I created a robots.txt file with the following. Note, need to allow /js/ to allow crawling to work correctly for pages using Plausible:
User-agent: *
Allow: /js/
Disallow: /
I then updated the docker-compose.yaml
adding a volumes mapping to this local file under the plausible service.
Lastly, restarted both Plausible and Apache and you should be well on your way to getting your Google Analytics alternative running.
$ sudo docker-compose down --remove-orphans && sudo docker-compose up -d
$ sudo /opt/bitnami/ctlscript.sh restart apache
All in all, the above took me less than a hour to get a working instance of Plausible.io up and running, self-hosted for $20/month on a 4GB Lightsail instance.
So far, I’ve been running this instance for 6 days and it looks pretty good. I’m getting a 500 error trying to import my old GA data so not quite sure what’s going on there but I did manage to get Google Search Console enabled but I’m not see where that data might show up. I’ve also setup email reporting and I’m curious to see what those look like.
Lastly, this project lives on its paid subscriptions to its cloud hosted version and assuming it works out I’ll be sure to use their sponsorship page.